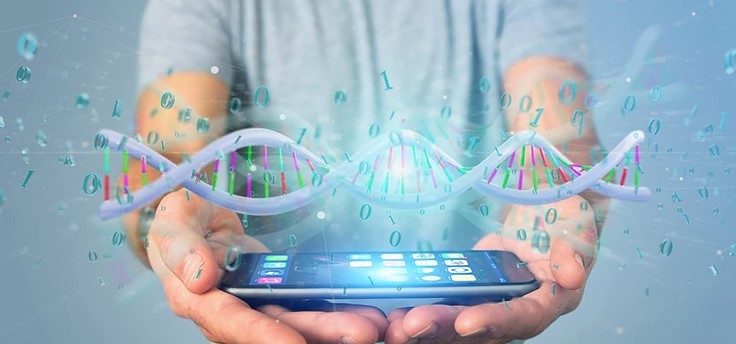
Dekel Taliaz
August, 2020//
The healthcare sector is buzzing with AI and Blockchain technology excitement. Yet, will they be our salvation or are they limiting our abilities to innovate? With research success driven by grants and published papers, are we learning from our failures – do we even know them? To truly innovate, we must ask those questions we don’t know how to solve. We must learn from our failures and invent new technologies – new ways to approach research! Let me explain more!
Innovation, “a new idea, method, or device”, is all about finding a need and then coming up with a new implementable solution to answer that need. However, all innovation begins by asking the right question.
The journalist Warren Berger, the author of A More Beautiful Question explains it perfectly:
“ …If you look at a lot of the innovations and breakthroughs today and you trace them back, as I did in my research, to their origin, a lot of times what you find at the root of it all is a great question; a beautiful question of someone asking why isn’t someone doing this or what if someone tried to do that? So I found that questions are often at the root of innovation.”
Almost by default, looking for ways to answer your question will force you to innovate.
Technology such as Artificial Intelligence (AI) lets us do this in new ways. However, we must not get blinded by the technology. We must never lose sight of what we are trying to achieve. What is our purpose? What is the question we are answering?
Follow the question and not the technology
Currently, Blockchain is being used in all sectors of the industry, from bitcoin, to tracking ownership or the provenance of documents, digital assets. The Blockchain revolution in the healthcare industry is just getting started. A study from IBM, found that 16% of surveyed healthcare executives had solid plans to implement a commercial Blockchain solution this year, while 56% expected to by 2020.
With so much buzz and excitement, it is not surprising that companies are looking to Blockchain technology to solve many problems. However, before starting out on any new innovation journey – whether it is for clinical research or commercial development – we should not be looking at the technology as a means to answer our question. Instead, we should be defining the question we are trying to solve, looking at how we can solve it and then building the right research design and hypothesis!
It is true existing technology provides us with ample opportunity to research existing data in new ways. As I have discussed in my previous blog, The future of clinical research: AI personalized, real-world studies, real world data and AI enable us to complete research in shorter ways at much less cost. We can now ask multiple questions that are constantly fine-tuned and optimized as we collect ongoing data.
Yet, true innovation – real technological breakthroughs – comes by asking those questions we often don’t know how to solve. Not looking at what we do know!
As Albert Einstein says,
“We cannot solve a problem by using the same kind of thinking we used when we created them.”
Understand technology limitations and focus on the purpose
To discover something new we must first ask ourselves what are we trying to find out? I might even go further and also say what interests us. Research requires drive, passion and grit! Most importantly, what is the need for this innovation – whether it be a new product or technology?
We must also understand the full limitations of the existing approaches and technologies when considering the “how” to answer our question. For example in the field of genetic sequencing, technology has opened up new ways to sequence the genome, yet approaches have their drawbacks. Let me explain some of the technology challenges we face at Taliaz, when trying to innovate a new personalized medicine approach in the complex genetics field of psychiatric disorders.
The drawbacks of genetic sequencing technologies for psychiatric disorders
Over a decade ago, the single nucleotide polymorphism (SNP) array was introduced, a type of DNA microarray which is used to detect polymorphisms within a population. It became almost a gold standard, with the platforms applications expanding. This then led to the trend of the Genome-wide association studies (GWAS), a way for scientists to identify single genetic associations with human disease.
The GWAS method searches the genome for small variations, called single nucleotide polymorphisms or SNPs (pronounced “snips”), which occur more frequently in people with a particular disease than in people without the disease. Each study can look at expression levels of tens of thousands of genes, and genotypes for millions of genetic markers (SNPs) can be measured, but each SNP association is determined by itself. This results in a large number of hypotheses tested at the same time, i.e. multiple simultaneous statistical tests or multiple comparisons, each of which has a potential to produce a genome “discovery.”
In genome sequencing, the Bonferroni correction, is the most common statistical method used to counteract the problem from multiple comparisons – the more conclusions we draw from the data, the more likely our conclusions are incorrect. However, the use of this statistical analysis has led to the loss of valuable information – as the correction comes at the cost of excluding relevant features which didn’t pass the robust statistical correction made. For GWAS, the consequences of multiple comparisons is widely considered to be one of the reasons for non-replication of study results.
Nevertheless, as would be expected in the psychiatric field, where the majority of disorders involve complex combinatorial genetics, analysis success with this approach has been limited.
The overuse of this technological approach has meant the rate of genetic discoveries in the mental health field has been slow.
Though these technologies have helped advance our understanding into our DNA, its pathophysiology and chronic conditions linked to genetics – there still remains a fundamental problem in the way we undertake research – limiting our capability to innovate.
The same thing is happening now with whole-exome sequencing (WES), a lower cost genomic technique for sequencing all of the protein-coding genes in a genome (known as the exome), which comprises roughly 1% of the whole genome. Though we have taken huge strides in sequencing the genome, our reliance on these technologies have left ~99% of the genome un-analyzed. Our research purpose in sequencing the gene has become reliant on the technology. For example, using this method makes us exclude regulatory elements from the analysis, which may be crucial for answering our research question.
Therefore, asking a question based on technology, and not looking for or even inventing new technologies which will allow us to answer our question, leaves many essential factors out of the hypothesized model.
Unfortunately, the way we are doing research is not just an issue for the genetic sector but in fact a growing problem for the whole research landscape.
One man’s research failure is another man’s research success
The research system as a whole, though invaluable, has some fundamental flaws.
We see that success in research today is measured by grants, awards and published papers. Personal and professional success – the recognition we seek and the way we view research centers of excellence – is often based on the number of peer-reviewed papers published and the size of the grants earned.
When we look at the content of those items that are published, we realize that over 85% of papers are only reporting the positive – the research that succeeded in achieving its goal. “Negative results” now account for only 14% of published papers, down from 30% in 1990. We are failing to learn from our research failures. When we look at the pioneers and major entrepreneurs of our time – Bill Gates, Henry Ford, Richard Branson – failure is part of success – in fact, it is strongly linked to innovation.
Furthermore, the majority of research studies cannot even be replicated. A rule of thumb among biotechnology venture-capitalists is that half of published research cannot be repeated.
Moreover, the scientific journals and academic publishing committees themselves are driving this spiraling success bias.
The most striking findings are the ones that have the greatest chance of making it onto the page. By constantly looking for new stories – interesting stories – innovative stories – we are missing the big research picture. We are choosing temporary excitement over true research findings. This is in fact representative of the news age we now live in.
Yet, for research to drive true innovation – we also need to approach the publishing and research follow-up in a new way – or even an old way! One that is systemic in its approach, to bring the same level of rigor used in producing the research evidence in the first place, to that in publishing our research.
Looking back at our past and learning from our beginnings is a good way. In the early 18th, 19th and even 20th century, research was a passion. The Nobel Prize winner, Marie Curie, is a great example of a scientific researcher innovating new technologies to answer a research question. Her scientific interest drove her in a very male dominated world, to invent the first mobile X-ray machine which helped to check the injured soldiers in the battlefield and the beginnings of the “atom” bomb.
Early published work of these research pioneers was as a means to share and communicate their findings. To help others learn from their research findings – to do better – to ask new questions. Success was measured very differently than today.
Clearly, going forward, we need to be promoting the sharing of knowledge – and most importantly our failures.
Remember – a failure to one researcher may actually be a success to another researcher when trying to answer a new or in some cases the same research question. If this information is not published – how will we know?!
Know your purpose, then find the technology – even invent it if you have to
I like to think this approach to research is our way at Taliaz. When developing our predictive AI algorithm, Predictix, to help clinicians find the right treatment for each of their patients sooner, we had a clear purpose. We were looking to find out how we can answer our research question – and trust me it was not an easy one!
What is key here is that we did not look at what technology and real world data were available and then try to come up with a research question. We had our research question already – how can we know which is the best antidepressant for each person. We came with the approach of looking at combinations of genetic alterations with clinical and environmental factors – and then we looked at how we could answer it.
As a result of our combinatorial hypothesis, we analyzed existing real-world data from patients suffering from depression in a whole new way. We used data available since 2008 from a collaborative study into depression by the National Institute of Mental Health – the STAR*D (Sequenced Treatment Alternatives to Relieve Depression) trial.
We then added in our theory – to truly personalize treatment we must understand genetic combinations and further combine them with a patient’s environmental and clinical background. In our scenario, the STAR*D real world data and AI were the technologies or tools to enable us to do that – our Predictix algorithm was our solution.
The future (and past) of research
It is clear that technology is advancing clinical research at epic rates. In the field of psychiatry, the digital revolution, AI and brain imaging are enabling us to discover and help treat psychiatric patients in ways 20 years ago, we could not fathom.
However, for research, especially brain research, to truly enter a new dimension we must celebrate our failures as much as our successes. We must use technology wisely and not lose focus of the purpose in why we are conducting the research.
Existing technologies such as AI and Blockchain – are breaking new ground never thought possible.
Yet, if we constantly focus on these and other emerging technologies to help us answer research questions just because they are available – we may miss the next big technological breakthrough.
Until next time! Dekel