Optimizing prediction of response to antidepressant medications using machine learning and environmental data
Spinrad, A., Darki-Morag, S. & Taliaz, D.
Optimizing prediction of response to antidepressant medications using machine learning and environmental data. Eur. Psychiatry 64, S755–S755 (2021).
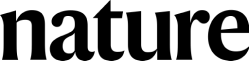
Summary
The current treatment for Major depression (MDD) consists mostly of trial and error, with antidepressants (AD) estimated to have response rates of 42%-53%. Combining clinical and demographic factors with feature selection techniques and machine-learning algorithms may contribute to understanding MDD’s multi factor complexity. Selected machine learning models for 5 antidepressants achieved a cumulative average accuracy of 60.12%.
Discover more about PREDICTIX Digital decision support tool.
Abstract
MDD is complex and multifactorial, presenting a major challenge to tailoring medication for each patient. Currently, treatment for MDD is mostly trial-and-error, with an estimated response rate of 42%-53% for antidepressants.
Objectives
We used a data-driven approach to analyze a combination of clinical and demographic factors in order to develop an accurate predictor of response to a panel of antidepressants and optimize treatment selection.
Methods
We analyzed the response patterns of patients to several antidepressant medications in the Sequenced Treatment Alternatives to Relieve Depression (STAR*D) study and the Pharmacogenomic Research Network Antidepressant Medication Pharmacogenomic Study (PGRN-AMPS), and employed state-of-the-art machine learning (ML) tools to generate a predictive algorithm. To validate our results and confirm the algorithm’s external generalizability outside of its training groups, we assessed its capacity to predict individualized antidepressant responses on a separate validation and test sets consisting of 1,021 patients overall from both studies.
Results
The algorithm’s ML prediction models achieved an average accuracy of 0.6416 (64.16%, SD 4.4) across the analyzed medications, and a cumulative accuracy of 0.6012 (60.12%), AUC of 0.601, sensitivity of 0.6034 (60.34%) and specificity of 0.599 (59.9%).
Conclusions
In this study, ML was applied to accumulating data derived from large studies in order to make a significant improvement in treating depression. Such predictive models might help doctors make better prescription decisions by analyzing a large amount of combinatorial data immediately on the spot, potentially enabling them to prescribe the right antidepressant medication earlier.